“TensorFlow Estimator”
Estimator
TensorFlow provides a higher level Estimator API with pre-built model to train and predict data.
It compose of the following steps:
Define the feature columns
In our example, we define a single feature with name f1.
import tensorflow as tf
import numpy as np
x_feature = tf.feature_column.numeric_column('f1')
We can use more than one feature. We can even pre normalize the feature with a lambda function:
n_room = tf.feature_column.numeric_column('n_rooms')
sqfeet = tf.feature_column.numeric_column('square_feet', normalizer_fn='lambda a: a - global_size')
input_fn
To import data to the Estimator later, we prepare an input_fn for training, testing and prediction respectively. In each input_fn, we provide all input features and values in x and the true labels in y.
# Training
train_input_fn = tf.estimator.inputs.numpy_input_fn(
x = {"f1": np.array([1., 2., 3., 4.])}, # Input features
y = np.array([1.5, 3.5, 5.5, 7.5]), # true labels
batch_size=2,
num_epochs=None, # Supply unlimited epochs of data
shuffle=True)
# Testing
test_input_fn = tf.estimator.inputs.numpy_input_fn(
x = {"f1": np.array([5., 6., 7.])},
y = np.array([9.5, 11.5, 13.5]),
num_epochs=1,
shuffle=False)
# Prediction
predict_input_fn = tf.estimator.inputs.numpy_input_fn(
x={"f1": np.array([8., 9.])},
num_epochs=1,
shuffle=False)
input_fn in general returns a tuple feature_dict and label. feature_dict is a dict containing the feature names and the feature data, and label contains the true values for all the samples.
def input_fn(dataset):
# manipulate dataset, extracting feature names and the label
# feature_dict : {"f1":..., "f2":...}
# label (y1, y2, ...)
return feature_dict, label
Use a pre-built estimator
TensorFlow comes with many built-in estimator:
- DNNClassifier
- DNNLinearCombinedClassifier
- DNNLinearCombinedRegressor
- DNNRegressor
- LinearClassifier
- LinearRegressor
To demonstrate the idea, we use the LinearRegressor to model:
\[y = Wx + b\]regressor = tf.estimator.LinearRegressor(
feature_columns=[x_feature],
model_dir='./output'
)
Training, validation and testing
Then we run the training, validation and testing with the corresponding input_fn.
regressor.train(input_fn=train_input_fn, steps=2500)
average_loss = regressor.evaluate(input_fn=test_input_fn)["average_loss"]
predictions = list(regressor.predict(input_fn=predict_input_fn))
We can print out the prediction by:
for input, p in zip(samples, predictions):
v = p["predictions"][0]
print(f"{input} -> {v:.4f}")
Source code
Here is the full program:
import tensorflow as tf
import numpy as np
x_feature = tf.feature_column.numeric_column('f1')
# Training
train_input_fn = tf.estimator.inputs.numpy_input_fn(
x = {"f1": np.array([1., 2., 3., 4.])}, # Input features
y = np.array([1.5, 3.5, 5.5, 7.5]), # true labels
batch_size=2,
num_epochs=None, # Supply unlimited epochs of data
shuffle=True)
# Testing
test_input_fn = tf.estimator.inputs.numpy_input_fn(
x = {"f1": np.array([5., 6., 7.])},
y = np.array([9.5, 11.5, 13.5]),
num_epochs=1,
shuffle=False)
# Prediction
samples = np.array([8., 9.])
predict_input_fn = tf.estimator.inputs.numpy_input_fn(
x={"f1": samples},
num_epochs=1,
shuffle=False)
regressor = tf.estimator.LinearRegressor(
feature_columns=[x_feature],
model_dir='./output'
)
regressor.train(input_fn=train_input_fn, steps=2500)
average_loss = regressor.evaluate(input_fn=test_input_fn)["average_loss"]
print(f"Average loss in testing: {average_loss:.4f}")
# Average loss in testing: 0.0000
predictions = list(regressor.predict(input_fn=predict_input_fn))
for input, p in zip(samples, predictions):
v = p["predictions"][0]
print(f"{input} -> {v:.4f}")
# 8.0 -> 15.4991
# 9.0 -> 17.4990
TensorBoard and checkpoint support
Estimator has built in support for checkpoint and TensorBoard. Checkpoints are saved automatically in model_dir. When we train the model again, model parameters will be reloaded from the checkpoint.
regressor = tf.estimator.LinearRegressor(
feature_columns=[x_feature],
model_dir='./output'
)
Also Estimator writes runtime information into the event logs in model_dir. To start the TensorBoard, we run the following command in a terminal and access the local server at port 6006.
tensorboard --logdir=output
It includes our loss during the training, the average lost in our validation and the number of iterations per second.
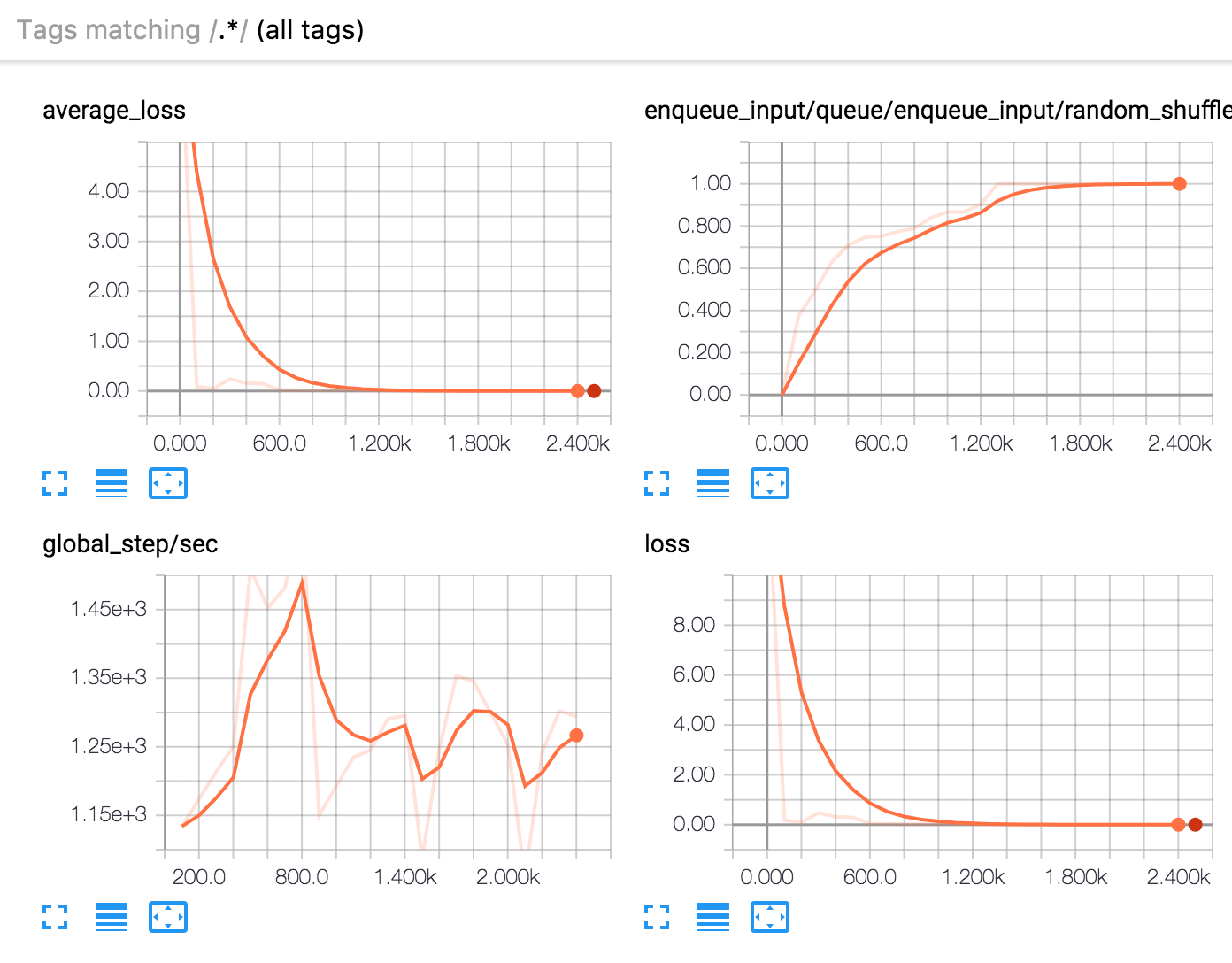
DNNClassifier
DNNClassifier is another pre-built estimator. We build a DNNClassifier with 2 hidden layers to classify the iris samples into 3 subclasses. We load 150 samples and split it into 120 training data and 30 testing data.
The iris dataset has 4 features: (image from wiki)
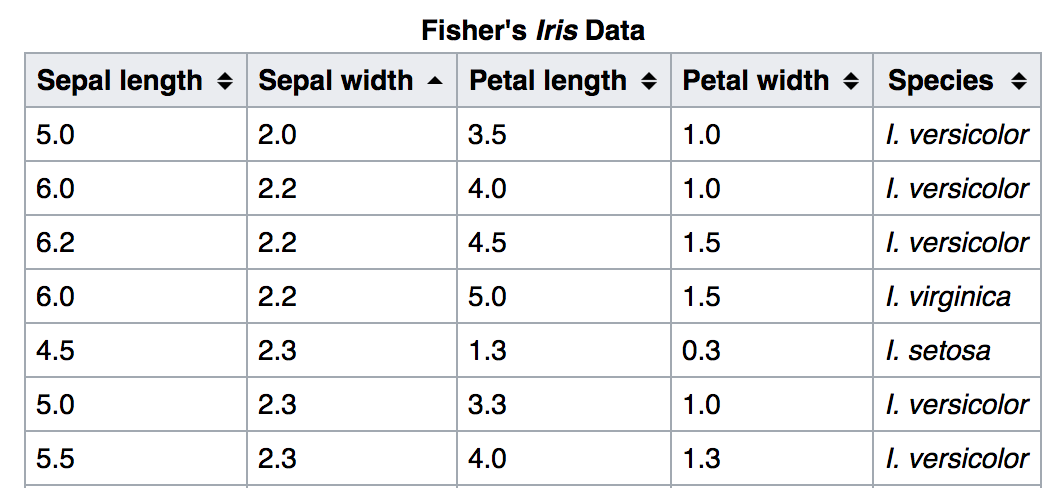
We first load the data for training and testing. We create a 4-feature column.
args = parser.parse_args(argv[1:])
# Fetch the Iris data and labels
# train_x shape: (120, 4), train_y (120,)
# test_x shape: (30, 4), test_y (30,)
(train_x, train_y), (test_x, test_y) = load_data()
# Feature columns describe how to use the input.
my_feature_columns = []
# x.keys = [SepalLength, SepalWidth, PetalLength, PetalWidth]
for key in train_x.keys():
my_feature_columns.append(tf.feature_column.numeric_column(key=key))
We then build a Deep neuron network classifier with 2 hidden layers with 10 units each. The model outputs a probability for each classes (3 classes) that we want to predict.
# Build a Deep Neuron Network
# with 2 hidden layers & 10, 10 units respectively, and
# classifying 3 output classes.
classifier = tf.estimator.DNNClassifier(
feature_columns=my_feature_columns,
hidden_units=[10, 10],
n_classes=3)
We train, test and predict the model with the corresponding input_fn:
# Train the Model.
classifier.train(
input_fn=lambda:train_input_fn(train_x, train_y, args.batch_size),
steps=args.train_steps)
# Evaluate the model.
eval_result = classifier.evaluate(
input_fn=lambda:eval_input_fn(test_x, test_y, args.batch_size))
print('\nTest set accuracy: {accuracy:0.3f}\n'.format(**eval_result))
# Test set accuracy: 0.967
# Generate predictions from the model
expected = ['Setosa', 'Versicolor', 'Virginica']
predict_x = {
'SepalLength': [5.1, 5.9, 6.9],
'SepalWidth': [3.3, 3.0, 3.1],
'PetalLength': [1.7, 4.2, 5.4],
'PetalWidth': [0.5, 1.5, 2.1],
}
predictions = classifier.predict(
input_fn=lambda:eval_input_fn(predict_x, labels=None, batch_size=args.batch_size))
In this example, the input_fn makes use of a Dataset to return the data:
def train_input_fn(features, labels, batch_size):
"""An input function for training"""
# Convert the inputs to a Dataset.
dataset = tf.data.Dataset.from_tensor_slices((dict(features), labels))
# Shuffle, repeat, and batch the examples.
dataset = dataset.shuffle(1000).repeat().batch(batch_size)
# Return the dataset.
return dataset
def eval_input_fn(features, labels, batch_size):
"""An input function for evaluation or prediction"""
features=dict(features)
if labels is None:
# No labels, use only features.
inputs = features
else:
inputs = (features, labels)
# Convert the inputs to a Dataset.
dataset = tf.data.Dataset.from_tensor_slices(inputs)
# Batch the examples
assert batch_size is not None, "batch_size must not be None"
dataset = dataset.batch(batch_size)
# Return the dataset.
return dataset
Finally we can print out the probability for each classes in our prediction:
for pred_dict, expec in zip(predictions, expected):
template = ('\nPrediction is "{}" ({:.1f}%), expected "{}"')
class_id = pred_dict['class_ids'][0]
probability = pred_dict['probabilities'][class_id]
print(template.format(SPECIES[class_id],
100 * probability, expec))
# Prediction is "Setosa" (99.9%), expected "Setosa"
# Prediction is "Versicolor" (99.7%), expected "Versicolor"
# Prediction is "Virginica" (95.5%), expected "Virginica"
Here is the complete code listing. We use pandas to read the csv data. For simplicity, we will let you to read the data loading code yourself.
import pandas as pd
TRAIN_URL = "http://download.tensorflow.org/data/iris_training.csv"
TEST_URL = "http://download.tensorflow.org/data/iris_test.csv"
CSV_COLUMN_NAMES = ['SepalLength', 'SepalWidth',
'PetalLength', 'PetalWidth', 'Species']
SPECIES = ['Setosa', 'Versicolor', 'Virginica']
def maybe_download():
train_path = tf.keras.utils.get_file(TRAIN_URL.split('/')[-1], TRAIN_URL)
test_path = tf.keras.utils.get_file(TEST_URL.split('/')[-1], TEST_URL)
return train_path, test_path
def load_data(y_name='Species'):
"""Returns the iris dataset as (train_x, train_y), (test_x, test_y)."""
train_path, test_path = maybe_download()
train = pd.read_csv(train_path, names=CSV_COLUMN_NAMES, header=0)
train_x, train_y = train, train.pop(y_name)
test = pd.read_csv(test_path, names=CSV_COLUMN_NAMES, header=0)
test_x, test_y = test, test.pop(y_name)
return (train_x, train_y), (test_x, test_y)
def train_input_fn(features, labels, batch_size):
"""An input function for training"""
# Convert the inputs to a Dataset.
dataset = tf.data.Dataset.from_tensor_slices((dict(features), labels))
# Shuffle, repeat, and batch the examples.
dataset = dataset.shuffle(1000).repeat().batch(batch_size)
# Return the dataset.
return dataset
def eval_input_fn(features, labels, batch_size):
"""An input function for evaluation or prediction"""
features=dict(features)
if labels is None:
# No labels, use only features.
inputs = features
else:
inputs = (features, labels)
# Convert the inputs to a Dataset.
dataset = tf.data.Dataset.from_tensor_slices(inputs)
# Batch the examples
assert batch_size is not None, "batch_size must not be None"
dataset = dataset.batch(batch_size)
# Return the dataset.
return dataset
# The remainder of this file contains a simple example of a csv parser,
# implemented using a the `Dataset` class.
# `tf.parse_csv` sets the types of the outputs to match the examples given in
# the `record_defaults` argument.
CSV_TYPES = [[0.0], [0.0], [0.0], [0.0], [0]]
def _parse_line(line):
# Decode the line into its fields
fields = tf.decode_csv(line, record_defaults=CSV_TYPES)
# Pack the result into a dictionary
features = dict(zip(CSV_COLUMN_NAMES, fields))
# Separate the label from the features
label = features.pop('Species')
return features, label
def csv_input_fn(csv_path, batch_size):
# Create a dataset containing the text lines.
dataset = tf.data.TextLineDataset(csv_path).skip(1)
# Parse each line.
dataset = dataset.map(_parse_line)
# Shuffle, repeat, and batch the examples.
dataset = dataset.shuffle(1000).repeat().batch(batch_size)
# Return the dataset.
return dataset
import argparse
import tensorflow as tf
parser = argparse.ArgumentParser()
parser.add_argument('--batch_size', default=100, type=int, help='batch size')
parser.add_argument('--train_steps', default=1000, type=int,
help='number of training steps')
def main(argv):
args = parser.parse_args(argv[1:])
# Fetch the Iris data and labels
# train_x shape: (120, 4), train_y (120,)
# test_x shape: (30, 4), test_y (30,)
(train_x, train_y), (test_x, test_y) = load_data()
# Feature columns describe how to use the input.
my_feature_columns = []
# x.keys = [SepalLength, SepalWidth, PetalLength, PetalWidth]
for key in train_x.keys():
my_feature_columns.append(tf.feature_column.numeric_column(key=key))
# Build a Deep Neuron Network
# with 2 hidden layers & 10, 10 units respectively, and
# classifying 3 output classes.
classifier = tf.estimator.DNNClassifier(
feature_columns=my_feature_columns,
hidden_units=[10, 10],
n_classes=3)
# Train the Model.
classifier.train(
input_fn=lambda:train_input_fn(train_x, train_y, args.batch_size),
steps=args.train_steps)
# Evaluate the model.
eval_result = classifier.evaluate(
input_fn=lambda:eval_input_fn(test_x, test_y, args.batch_size))
print('\nTest set accuracy: {accuracy:0.3f}\n'.format(**eval_result))
# Test set accuracy: 0.967
# Generate predictions from the model
expected = ['Setosa', 'Versicolor', 'Virginica']
predict_x = {
'SepalLength': [5.1, 5.9, 6.9],
'SepalWidth': [3.3, 3.0, 3.1],
'PetalLength': [1.7, 4.2, 5.4],
'PetalWidth': [0.5, 1.5, 2.1],
}
predictions = classifier.predict(
input_fn=lambda:eval_input_fn(predict_x, labels=None, batch_size=args.batch_size))
for pred_dict, expec in zip(predictions, expected):
template = ('\nPrediction is "{}" ({:.1f}%), expected "{}"')
class_id = pred_dict['class_ids'][0]
probability = pred_dict['probabilities'][class_id]
print(template.format(SPECIES[class_id],
100 * probability, expec))
# Prediction is "Setosa" (99.9%), expected "Setosa"
# Prediction is "Versicolor" (99.7%), expected "Versicolor"
# Prediction is "Virginica" (95.5%), expected "Virginica"
if __name__ == '__main__':
tf.logging.set_verbosity(tf.logging.INFO)
tf.app.run(main)
Custom model for Estimator
TensorFlow allows us to build custom models for estimators. We need to implement a function for model_fn to build our custom model, loss function, evaluation matrices
- build a network with 2 hidden layers and one output layer.
- calculate the predicted class
- compute the loss function
- add evaluation metrics to be displayed in the TensorBoard
- create optimizer and trainer
def my_model(features, labels, mode, params):
"""DNN with 2 hidden layers."""
# features is a dict with elements containing "feature name": values
# labels contains the true labels for the data. Shape (?. )
# mode: train, eval or infer.
# params =
# {'feature_columns':
# [_NumericColumn(key='SepalLength', shape=(1,), default_value=None, dtype=tf.float32, normalizer_fn=None),
# _NumericColumn(key='SepalWidth', shape=(1,), default_value=None, dtype=tf.float32, normalizer_fn=None),
# _NumericColumn(key='PetalLength', shape=(1,), default_value=None, dtype=tf.float32, normalizer_fn=None),
# _NumericColumn(key='PetalWidth', shape=(1,), default_value=None, dtype=tf.float32, normalizer_fn=None)],
# 'hidden_units': [10, 10],
# 'n_classes': 3}
# Build the network
net = tf.feature_column.input_layer(features, params['feature_columns'])
# Create 2 hidden layers with 10 units each
for units in params['hidden_units']:
net = tf.layers.dense(net, units=units, activation=tf.nn.relu)
# Compute logits (1 per class).
logits = tf.layers.dense(net, params['n_classes'], activation=None)
### Compute predictions.
predicted_classes = tf.argmax(logits, 1)
# Return if it is in prediction mode
if mode == tf.estimator.ModeKeys.PREDICT:
predictions = {
'class_ids': predicted_classes[:, tf.newaxis],
'probabilities': tf.nn.softmax(logits),
'logits': logits,
}
return tf.estimator.EstimatorSpec(mode, predictions=predictions)
### Compute loss.
loss = tf.losses.sparse_softmax_cross_entropy(labels=labels, logits=logits)
### Compute evaluation metrics.
accuracy = tf.metrics.accuracy(labels=labels,
predictions=predicted_classes,
name='acc_op')
metrics = {'accuracy': accuracy}
tf.summary.scalar('accuracy', accuracy[1])
# Return if in evaluation mode
if mode == tf.estimator.ModeKeys.EVAL:
return tf.estimator.EstimatorSpec(
mode, loss=loss, eval_metric_ops=metrics)
### Create optimizer and trainer
assert mode == tf.estimator.ModeKeys.TRAIN
# Create the optimizer
optimizer = tf.train.AdagradOptimizer(learning_rate=0.1)
train_op = optimizer.minimize(loss, global_step=tf.train.get_global_step())
return tf.estimator.EstimatorSpec(mode, loss=loss, train_op=train_op)
To create a custom Estimator:
# Build 2 hidden layer DNN with 10, 10 units respectively.
classifier = tf.estimator.Estimator(
model_fn=my_model,
params={
'feature_columns': my_feature_columns,
# Two hidden layers of 10 nodes each.
'hidden_units': [10, 10],
# The model must choose between 3 classes.
'n_classes': 3,
})
Here is the full source code. However, we will skip the part in reading iris dataset since it is the same as the previous example:
import argparse
import tensorflow as tf
import pandas as pd
parser = argparse.ArgumentParser()
parser.add_argument('--batch_size', default=100, type=int, help='batch size')
parser.add_argument('--train_steps', default=1000, type=int,
help='number of training steps')
def my_model(features, labels, mode, params):
"""DNN with 2 hidden layers."""
# features is a dict with elements containing "feature name": values
# labels contains the true labels for the data. Shape (?. )
# mode: train, eval or infer.
# params =
# {'feature_columns':
# [_NumericColumn(key='SepalLength', shape=(1,), default_value=None, dtype=tf.float32, normalizer_fn=None),
# _NumericColumn(key='SepalWidth', shape=(1,), default_value=None, dtype=tf.float32, normalizer_fn=None),
# _NumericColumn(key='PetalLength', shape=(1,), default_value=None, dtype=tf.float32, normalizer_fn=None),
# _NumericColumn(key='PetalWidth', shape=(1,), default_value=None, dtype=tf.float32, normalizer_fn=None)],
# 'hidden_units': [10, 10],
# 'n_classes': 3}
# Build the network
net = tf.feature_column.input_layer(features, params['feature_columns'])
# Create 2 hidden layers with 10 units each
for units in params['hidden_units']:
net = tf.layers.dense(net, units=units, activation=tf.nn.relu)
# Compute logits (1 per class).
logits = tf.layers.dense(net, params['n_classes'], activation=None)
### Compute predictions.
predicted_classes = tf.argmax(logits, 1)
# Return if it is in prediction mode
if mode == tf.estimator.ModeKeys.PREDICT:
predictions = {
'class_ids': predicted_classes[:, tf.newaxis],
'probabilities': tf.nn.softmax(logits),
'logits': logits,
}
return tf.estimator.EstimatorSpec(mode, predictions=predictions)
### Compute loss.
loss = tf.losses.sparse_softmax_cross_entropy(labels=labels, logits=logits)
### Compute evaluation metrics.
accuracy = tf.metrics.accuracy(labels=labels,
predictions=predicted_classes,
name='acc_op')
metrics = {'accuracy': accuracy}
tf.summary.scalar('accuracy', accuracy[1])
# Return if in evaluation mode
if mode == tf.estimator.ModeKeys.EVAL:
return tf.estimator.EstimatorSpec(
mode, loss=loss, eval_metric_ops=metrics)
### Create optimizer and trainer
assert mode == tf.estimator.ModeKeys.TRAIN
# Create the optimizer
optimizer = tf.train.AdagradOptimizer(learning_rate=0.1)
train_op = optimizer.minimize(loss, global_step=tf.train.get_global_step())
return tf.estimator.EstimatorSpec(mode, loss=loss, train_op=train_op)
def main(argv):
args = parser.parse_args(argv[1:])
# Fetch the data
(train_x, train_y), (test_x, test_y) = load_data()
# Feature columns describe how to use the input.
my_feature_columns = []
for key in train_x.keys():
my_feature_columns.append(tf.feature_column.numeric_column(key=key))
# Build 2 hidden layer DNN with 10, 10 units respectively.
classifier = tf.estimator.Estimator(
model_fn=my_model,
params={
'feature_columns': my_feature_columns,
# Two hidden layers of 10 nodes each.
'hidden_units': [10, 10],
# The model must choose between 3 classes.
'n_classes': 3,
})
# Train the Model.
classifier.train(
input_fn=lambda:train_input_fn(train_x, train_y, args.batch_size),
steps=args.train_steps)
# Evaluate the model.
eval_result = classifier.evaluate(
input_fn=lambda:eval_input_fn(test_x, test_y, args.batch_size))
# Generate predictions from the model
expected = ['Setosa', 'Versicolor', 'Virginica']
predict_x = {
'SepalLength': [5.1, 5.9, 6.9],
'SepalWidth': [3.3, 3.0, 3.1],
'PetalLength': [1.7, 4.2, 5.4],
'PetalWidth': [0.5, 1.5, 2.1],
}
predictions = classifier.predict(
input_fn=lambda:eval_input_fn(predict_x,
labels=None,
batch_size=args.batch_size))
if __name__ == '__main__':
tf.logging.set_verbosity(tf.logging.INFO)
tf.app.run(main)
Estimator using Layer module and Logging hook
Logging hook allows us to log addition information to the log output. LoggingTensorHook indicates the Tensors that we want to log in computing the prediction.
tf.logging.set_verbosity(tf.logging.INFO)
def cnn_model_fn(features, labels, mode):
...
logits = tf.layers.dense(inputs=dropout, units=10)
predictions = {
"classes": tf.argmax(input=logits, axis=1),
"probabilities": tf.nn.softmax(logits, name="softmax_tensor")
}
def main(unused_argv):
...
mnist_classifier = tf.estimator.Estimator(model_fn=cnn_model_fn,
model_dir="/tmp/mnist_convnet_model")
tensors_to_log = {"probabilities": "softmax_tensor"}
logging_hook = tf.train.LoggingTensorHook(tensors=tensors_to_log, every_n_iter=50)
We also build a new model using tf.layers.
def cnn_model_fn(features, labels, mode):
input_layer = tf.reshape(features["x"], [-1, 28, 28, 1])
conv1 = tf.layers.conv2d(inputs=input_layer, filters=32, kernel_size=[5, 5], padding="same", activation=tf.nn.relu)
pool1 = tf.layers.max_pooling2d(inputs=conv1, pool_size=[2, 2], strides=2)
conv2 = tf.layers.conv2d(inputs=pool1, filters=64, kernel_size=[5, 5], padding="same", activation=tf.nn.relu)
pool2 = tf.layers.max_pooling2d(inputs=conv2, pool_size=[2, 2], strides=2)
pool2_flat = tf.reshape(pool2, [-1, 7 * 7 * 64])
dense = tf.layers.dense(inputs=pool2_flat, units=1024, activation=tf.nn.relu)
dropout = tf.layers.dropout(inputs=dense, rate=0.4, training=mode == tf.estimator.ModeKeys.TRAIN)
logits = tf.layers.dense(inputs=dropout, units=10)
Here is the full source code
import numpy as np
import tensorflow as tf
tf.logging.set_verbosity(tf.logging.INFO)
def cnn_model_fn(features, labels, mode):
input_layer = tf.reshape(features["x"], [-1, 28, 28, 1])
conv1 = tf.layers.conv2d(inputs=input_layer, filters=32, kernel_size=[5, 5], padding="same", activation=tf.nn.relu)
pool1 = tf.layers.max_pooling2d(inputs=conv1, pool_size=[2, 2], strides=2)
conv2 = tf.layers.conv2d(inputs=pool1, filters=64, kernel_size=[5, 5], padding="same", activation=tf.nn.relu)
pool2 = tf.layers.max_pooling2d(inputs=conv2, pool_size=[2, 2], strides=2)
pool2_flat = tf.reshape(pool2, [-1, 7 * 7 * 64])
dense = tf.layers.dense(inputs=pool2_flat, units=1024, activation=tf.nn.relu)
dropout = tf.layers.dropout(inputs=dense, rate=0.4, training=mode == tf.estimator.ModeKeys.TRAIN)
logits = tf.layers.dense(inputs=dropout, units=10)
predictions = {
"classes": tf.argmax(input=logits, axis=1),
"probabilities": tf.nn.softmax(logits, name="softmax_tensor")
}
if mode == tf.estimator.ModeKeys.PREDICT:
return tf.estimator.EstimatorSpec(mode=mode, predictions=predictions)
onehot_labels = tf.one_hot(indices=tf.cast(labels, tf.int32), depth=10)
loss = tf.losses.softmax_cross_entropy(onehot_labels=onehot_labels, logits=logits)
if mode == tf.estimator.ModeKeys.TRAIN:
optimizer = tf.train.GradientDescentOptimizer(learning_rate=0.001)
train_op = optimizer.minimize(loss=loss, global_step=tf.train.get_global_step())
return tf.estimator.EstimatorSpec(mode=mode, loss=loss, train_op=train_op)
eval_metric_ops = {
"accuracy": tf.metrics.accuracy(
labels=labels, predictions=predictions["classes"])}
return tf.estimator.EstimatorSpec(mode=mode, loss=loss,
eval_metric_ops=eval_metric_ops)
def main(unused_argv):
mnist = tf.contrib.learn.datasets.load_dataset("mnist")
train_data = mnist.train.images
train_labels = np.asarray(mnist.train.labels, dtype=np.int32)
eval_data = mnist.test.images
eval_labels = np.asarray(mnist.test.labels, dtype=np.int32)
mnist_classifier = tf.estimator.Estimator(model_fn=cnn_model_fn,
model_dir="/tmp/mnist_convnet_model")
tensors_to_log = {"probabilities": "softmax_tensor"}
logging_hook = tf.train.LoggingTensorHook(tensors=tensors_to_log, every_n_iter=50)
# Train the model
train_input_fn = tf.estimator.inputs.numpy_input_fn(x={"x": train_data},
y=train_labels, batch_size=100, num_epochs=None, shuffle=True)
mnist_classifier.train(input_fn=train_input_fn,
steps=20000, hooks=[logging_hook])
# Evaluate the model and print results
eval_input_fn = tf.estimator.inputs.numpy_input_fn(x={"x": eval_data},
y=eval_labels, num_epochs=1, shuffle=False)
eval_results = mnist_classifier.evaluate(input_fn=eval_input_fn)
print(eval_results)
if __name__ == "__main__":
tf.app.run()